Featured Book
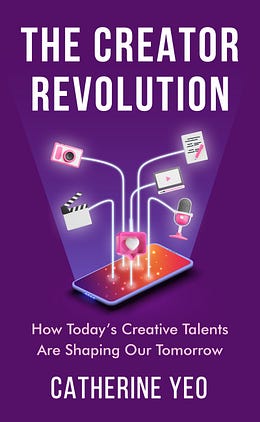
The Creator Revolution: How Today’s Creative Talents Are Shaping Our Tomorrow
The Creator Revolution: How Today’s Creative Talents Are Shaping Our Tomorrow
The Creator Revolution examines the historic rise of digital content creators and their impact on transforming both our present and our future.
2022
Catherine Yeo
Catherine Yeo