ChatGPT Reality Check: Why Transformative AI Takes Time
Rome wasn’t built in a day, and neither is AI
Undoubtedly, ChatGPT and other LLMs represent a paradigm shift in technology. I don’t deny this fact. Yet, on this forum, it’s not uncommon to encounter assertions such as “x industry will vanish by 2024” or “in 6 months, every obstacle will be conquered,” which are treated as truth despite their speculative nature.
Join the Medium Membership Program for only 5$ to continue learning without limits. I’ll receive a small portion of your membership fee if you use the following link, at no extra cost to you.
Recognizing that LLMs might ultimately have the profound impact many anticipate (I believe they will, given time), let’s explore several reasons why this shift won’t transpire as quickly as some expect. I’ll touch upon the technological boundaries, which are comparatively simple to dissect, before delving into the crux of the matter.
To be honest, I’m not suggesting that these challenges are insurmountable or even improbable to overcome. My focus lies on the velocity of change and the aspects that will impede its progress.
In a nutshell: Although LLMs are poised to revolutionize our world, significant transformations don’t occur overnight.
Technological Constraints
- At their core, generative AIs are designed to create content. The real essence of most jobs lies not in the content itself but in the decision-making process. This involves asking the right questions, consulting the appropriate individuals, and relying on trustworthy sources. Until AI can take substantial initiative, it will primarily serve as an instrument for more capable human employees.
- When it comes to content creation, ChatGPT might outperform the average person in many tasks, but it falls short when compared to experts in their respective fields. The technology might have reached 80% of its potential, but the remaining 20% poses a significant challenge, particularly in areas like law and medicine where accuracy is crucial.
- While ChatGPT’s immediate applications seem to lie in the tech industry and other “hard” fields, with developers as its most eager early adopters, the majority of the economy lies outside of STEM. A considerable portion of the economy revolves around sectors such as trades and care services, where the output cannot be replaced by AI. Even in industries that appear ripe for disruption, the reality can be more complex. For instance, in the public policy sector where I work, ChatGPT is not advanced enough to replace the majority of tasks, mainly because policy work depends on intangible factors like political connections, societal consequences, and public sentiment.
Economic Challenges
- Although ChatGPT might theoretically possess the capacity to supplant workers in certain situations, financial and logistical considerations frequently render it too costly or intricate. Many organizations lack the necessary expertise and may not acquire it in the near future. For instance, numerous small businesses are still struggling to fully utilize the internet. While ChatGPT can handle customer inquiries, building the infrastructure required for it to be a cost-saving investment is another matter altogether.
- Similarly, autonomous robotics is presently not economically viable for most real-world applications. The significant capital expenditure and logistical hurdles associated with manufacturing and distribution imply that adoption will be gradual. As a result, the majority of low-wage service workers will likely retain their jobs for the time being.
- Elaborating on these ideas, the widespread implementation of technology often incurs massive expenses in both capital and human resources. It took some of the largest companies and governments around the globe approximately two decades to thoroughly incorporate the internet into their operations, let alone personal computers. Even if a CEO is keen on employing AI for functions like accounting, HR, or marketing, it demands considerable time to create effective systems, especially when the technology is novel and lacks established guidelines to follow.
Socio-cultural Obstacles
- Legal liability poses a significant challenge for companies and governments. Regardless of how advanced the technology becomes, unresolved concerns about reliability and legitimacy will prevent LLMs from being assigned tasks that could legally compromise these entities.
- Privacy is another crucial concern, and not just for the more apparent cases like banks safeguarding personal information. In regions like the EU, which enforce strict data privacy regulations, many applications may not permit the use of LLMs, particularly if requests are processed through U.S. servers.
- Cultural resistance is also a factor, and not just the neo-Luddite reactions seen in some governments. Generally, decision-makers in staffing are older and more skeptical of emerging technologies. It will take considerable time for numerous managers to feel comfortable replacing human staff with untested tech alternatives. It’s worth mentioning that many parts of the world are not as competitive as the U.S. tech industry, where employers and governments intervene to protect workers.
- While I’ve mentioned privacy regulations, labor laws and other rules also play a role. Unionized workplaces won’t accept changes without resistance, and we can expect new regulations limiting AI usage.
- Perhaps most importantly, people have a natural affinity for human interaction. In subjective domains like art and commentary, many individuals will continue to favor human work even if AI-produced content is virtually indistinguishable. For instance, Stephen King will likely outsell an AI version of Mark Twain. On a smaller scale, people will still listen to local radio hosts, attend local colleges, and hire local marketing firms due to the human connection. The cultural acceptance of AI-generated content will be a protracted battle.
To drive my cat home, I’m not suggesting that LLMs like ChatGPT won’t have significant impacts or that these challenges won’t be eventually overcome. My point is that due to these obstacles, the transformation might not happen as rapidly as some people anticipate or fear.
One great example that encapsulates all these points is the librarian profession. It was predicted that search engines would make librarians obsolete, as computers could seemingly perform their primary tasks more efficiently. Nevertheless, the number of librarians today has mostly stayed constant. This can be attributed to the following factors:
- Librarians perform tasks beyond just finding and cataloging resources, as they also assess the significance of information.
- Hiring a librarian continues to be more cost-effective than deploying automated book-shelving robots.
- Librarians offer a warm, human presence that people value.
My other writings —

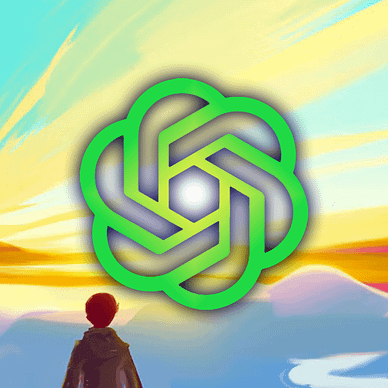
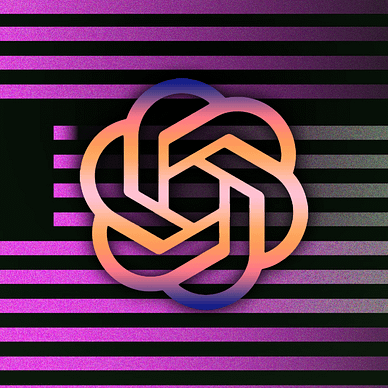